ISTE Standards for Coaches 6b, “Support educators to interpret qualitative and quantitative data to inform their decisions and support individual student learning,” reminds educational leaders that educators need support in not only gathering data but accurately analyzing that data so that informed best-practice decisions for instruction can be made and implemented.
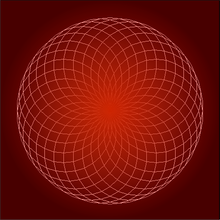
Source: EDTC 6106 Program Evaluation Project, Seattle Pacific University
Evidence: “Careful analysis and coding of responses yielded six themes for data analysis: breakout rooms, content & pacing, face-to-face versus virtual, positive, not applicable, and technology related. Additional correlation of subgroup mean referral scores organized by theme were reviewed relative to overall scores.”
Evidence: “The prevalence of each theme warrants consideration of factors driving participants feedback and improvements to be made. Additional analysis of subgroup correlations to overall mean referral scores yielded design insights worth pursuing and informed possibilities for improving participants’ overall experience.”
Evidence: “The goals of this research analysis were to review survey feedback from Digital Experience Math Community of Practice participants to help the professional learning design team determine what aspects of the professional learning experience worked well, what needed to change, and where improvements could be made. Sorting through over 450 answers proved challenging as answers were extremely varied but some key overarching patterns emerged. These were coded into general topics relating to the comments themselves. These topics were then used to develop six overarching themes that could be used to categorize participant responses. Responses were then coded into one of these theme categories which allowed for more detailed analysis.”
Explanation: Educators need support to effectively analyze data in a formal, research-based manner. This becomes even more important when conducting the data collection at relatively large scales. Initial analysis as to whether data is qualitative or quantitative will help support educator understanding of what the collected results may mean. Additionally, support with coding, looking for patterns, and statistical analysis can help educators make more effectively informed decisions with regard to supporting individual student learning. The six themes identified for data analysis in this project can serve as a good example of how one might approach this type of project. Please see the table below for a corresponding explanation of each theme.
THEME | DESCRIPTION |
Breakout Rooms | Responses generally described how participants felt breakout rooms related to and affected their overall experience. |
Content & Pacing | Responses centered on either quantity of content or speed of pacing and were grouped together due to the interdependence of these two factors. |
Face-to-Face versus Virtual | Responses focused on comparing and contrasting in-person versus online training with most focusing on the advantages of being in-person versus the challenges of learning remotely. |
Positive | Responses overwhelmingly emphasized positive aspects of the training with little mention of other factors. |
Not Applicable | Responses either consisted of an “NA” response, were difficult to discern the meaning given the context, or otherwise unintelligible. |
Technology Related | Responses primarily identified technology related factors in the feedback and how the utilization of various technologies impacted the training. |
The ability to code for patterns in qualitative feedback supports the general educator’s ability to practice more targeted statistical analysis. While the data from this project was extensive and is not publicly available for publishing online, it is safe to utilize a generic categorical comparison as an example. The overall average net promoter provided a general bar against which data categories could be disaggregated, compared, and studied. For example, the average net promoter score (on a scale of 1-10) of participant responses labeled “Technology Related” was lower than the overall average net promoter score by a value of 1.1. This is very significant and tells designers of the course that finding ways to address, minimize, and smooth out technology challenges could lead to significant increases in the overall net promoter score for this course. Such lessons learned teach a lot about the potential of data analysis and can be applied in general to other projects involving qualitative analysis.
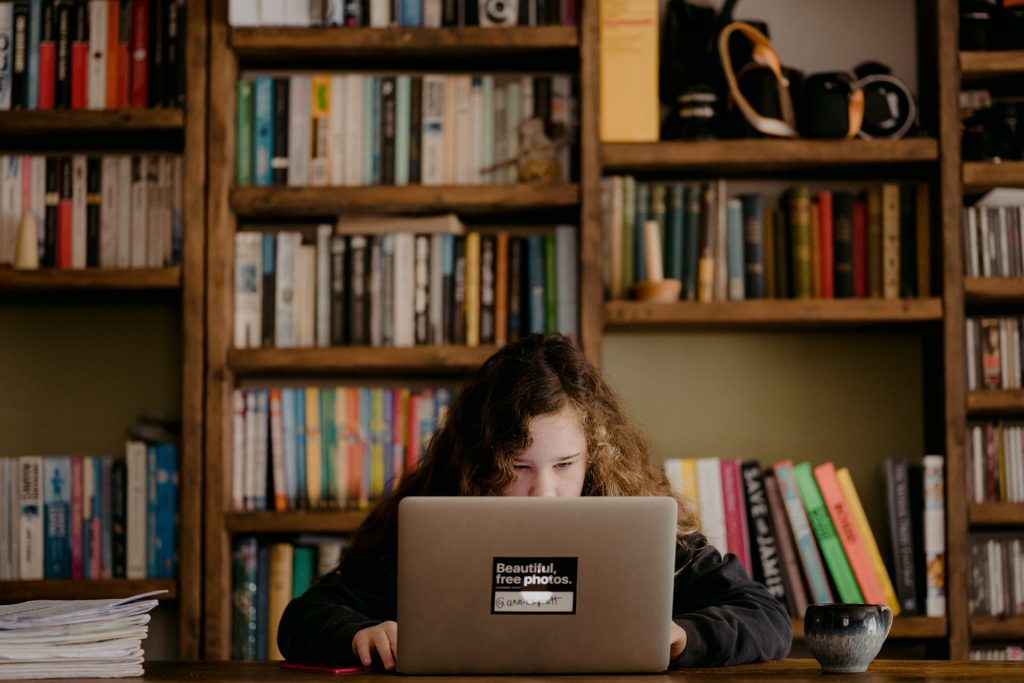